Promptlink’s Labeled Data Products are comprehensive, high-quality, and well-defined sets of Labeled Data for use as Machine-Learning (ML) training data in various AI-based applications in broadband network operations.
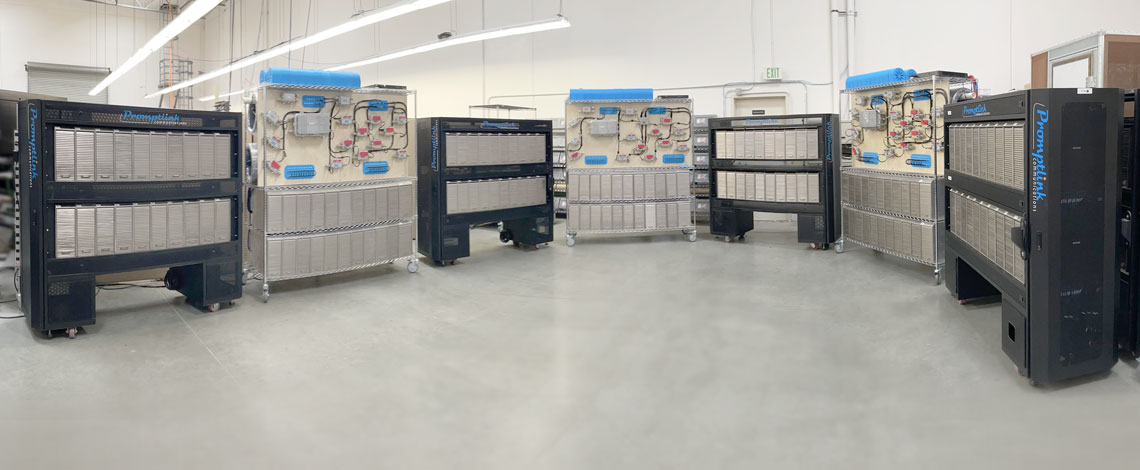
The datasets are collected from a real Hybrid Fiber Coaxial (HFC) Network constructed in a controlled lab environment and labeled according to different network conditions. This is achieved by inserting multiple real-world, impaired network components at different locations within the HFC network and introducing many different types of noise and distortions into the network environment. The result is a flexible set of Labeled Data in correspondence with well-defined and precisely placed impaired component(s) and impairments. This unique dataset can be used to feed Machine-Learning applications for training or verification purposes to make them fully capable of their usage.
In this way, network operators can precisely match field findings with these datasets that accurately reproduce the specific network components and conditions found in a specific network.
The lab has the ultimate flexibility to be reconfigured to meet any needs and requirements that may be desired.
Lab Setup
The Data Labeling Lab setup includes a simulated RF network featuring fiber nodes, various models of CMTS, various CPE, and other network components. To ensure immunity from external noise, cross-neighbor interference and to prevent noise emission, the modems are housed within shielded enclosures. Similarly, the impairments and noise sources, which are intended to serve as labels, are also placed in shielded enclosures to isolate them from outside environmental noise. Finally, a labeling software application is employed to automatically control and apply different noise sources while simultaneously collecting and labeling data.
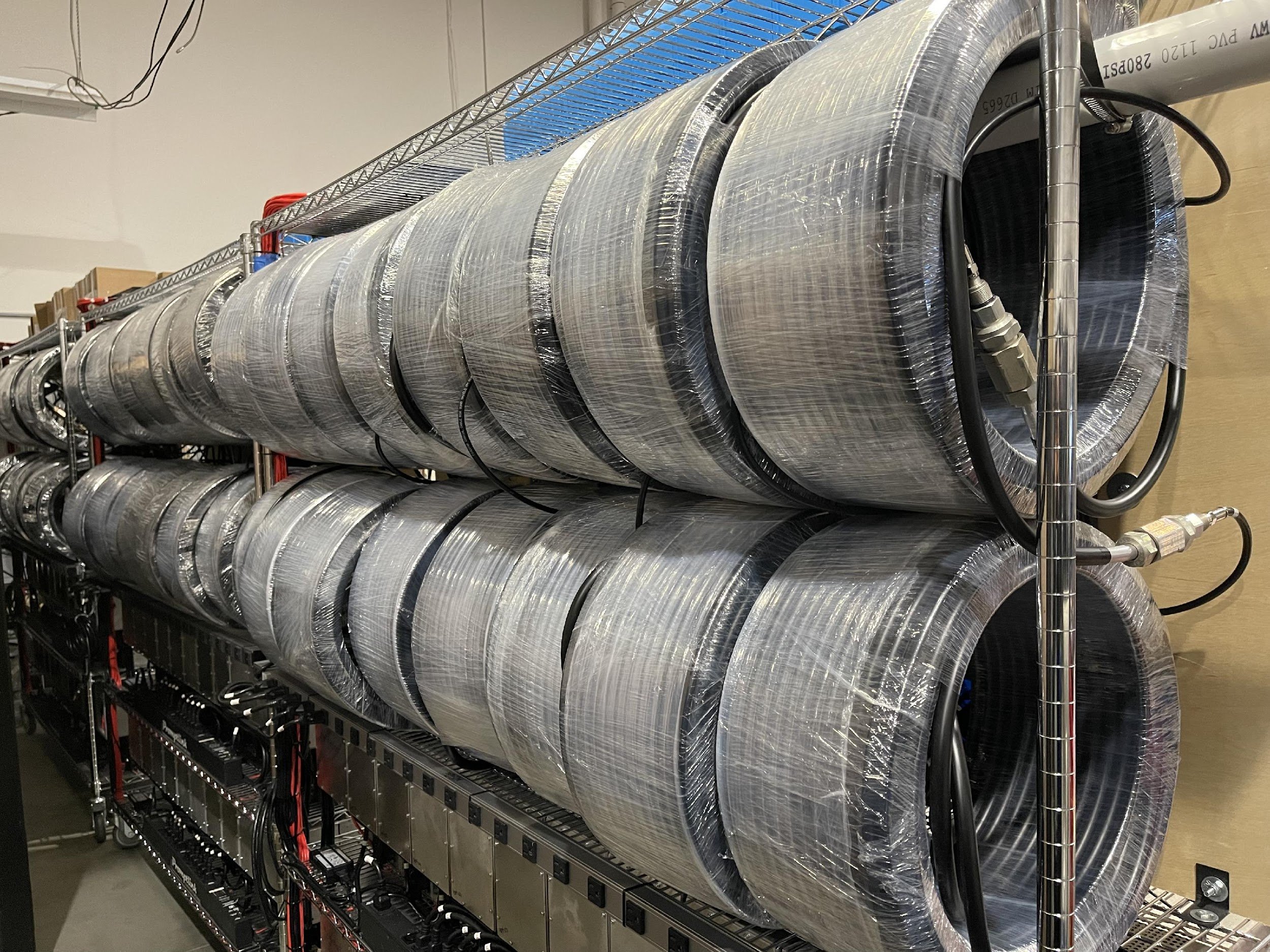
Figure 1 - Rolls of trunk line allow for distance calculation.
How is the Lab hardware configured?
The Promptlink Noise Lab can be configured for multiple uses. For example, the Data Labeling Lab setup includes a simulated RF network featuring fiber nodes, various models of CMTS, various CPE, and other network components. To ensure immunity from external noise, cross-neighbor interference and to prevent noise emission, the modems are housed within shielded enclosures. Similarly, the impairments and noise sources, which are intended to serve as labels, are also placed in shielded enclosures to isolate them from outside environmental noise. Finally, a labeling software application is employed to automatically control and apply different noise sources while simultaneously collecting and labeling data.
As mentioned, the lab can be configured for multiple uses beyond usage for Data Labeling. Data Labeling is a configuration designed both for use in Promptlink’s Network NoiseHawkAI (add link) and for use with MSO-designed Machine Learning engines.
Impaired Network Components Characterization Lab
Used for measuring the transmission line parameters and characterizing a specific impaired network component
Noise Source
Characterization Lab
Used for measuring the noise statistics at different frequencies and characterizing different noise sources.
Corroded and Damaged Network Components Leakage and Ingress Characterization Lab
Used for measuring the leakage and Ingress at different frequencies and characterizing a faulty/damaged network component.
Noise Spectral Density Calibration Lab
Used to develop calibration tables for calculating downstream Noise Spectral Density at the cable modem from MER and Power Level for different makes and model cable modems.
The utilization of Faraday Cages for larger impairments and Shielded Enclosures for individual modems provides confinement and isolation of the unintended noise generated by each modem. This setup facilitates realistic simulation of noise impairments, including those from external sources like home appliances and industrial machinery, when there is a failed or impaired network component. Additionally, shielding ensures that intentionally generated noise only affects the intended area of the network, isolating it from other sections and their components.
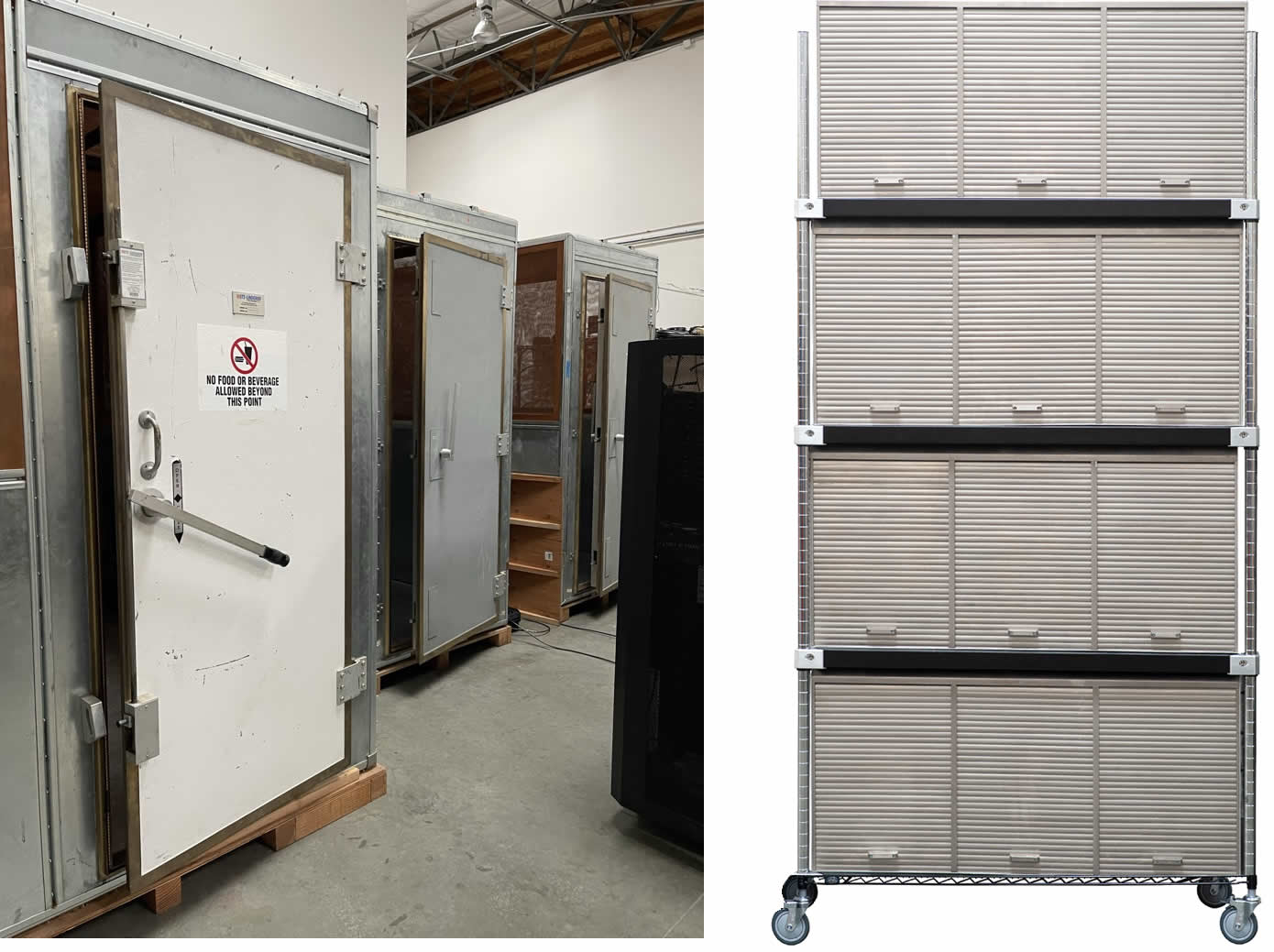
Figure 2 – Large and Small Faraday Cages Allow for Isolation of RF Noise.
As an example, to facilitate Data Labeling, all racks are meticulously configured to match one another. Identical modems are positioned in corresponding spots within both the network segments and MDU racks, with specified and matching power levels. For instance, each of the four MDU racks' slot one contains identical modems with the same power levels. While the modems in other slots are different from those in slot one of each MDU rack, consistency is maintained across the same slot in different racks. This approach enables the captured and labeled data to be utilized for studying timing offset variations across different parameters related to cable modems, including modem types at four different distances. Furthermore, when impaired components and noise are introduced at various network locations, the effects of noise and impairments on telemetry data, including timing offsets, can be captured. This comprehensive set of labeled data serves not only for noise and impairment localization but also as training data for automated prediction and extraction of the network topology. Collected data consists of all DOCSIS and PNM monitoring parameters.
Noise Modeling, Characterization and Cataloging
In the real world, RF noise originates from diverse sources such as household appliances, industrial machinery, and lighting, among others. Each source exhibits unique noise characteristics or signatures. These characteristics can vary widely, including impulse noise, white noise, and noise that is more pronounced at specific frequencies. The nature of noise can be steady, fluctuating over time, or sporadic. In the realm of noise localization applications using Machine-Learning techniques, accurate Data Labeling for different types of noise is crucial, necessitating the use of parametric models. Generic labels like 'noise from a desk lamp' are inadequate due to the significant variation in noise signatures, even among similar items. Therefore, the emphasis in Data Labeling must be on the specific noise signature rather than the general source. The aim of this effort is to develop mathematical models for various noise types and to experimentally characterize a wide range of noise sources in a test setup. These characterized noise sources will then be utilized in our Data Labeling processes.
A suitable model for noise characterization, applicable in Data Labeling for training noise localization ML models, involves using statistical parameters for different frequency bands of the noise spectrum. This is because the noise spectrum can dynamically change or remain static over time for different frequency bands of the spectrum. In this model, the defining parameters are the maximum, minimum, and mean spectrum of noise, as well as the standard deviation of noise power at different frequencies.
Examples of Various Noise Characteristics
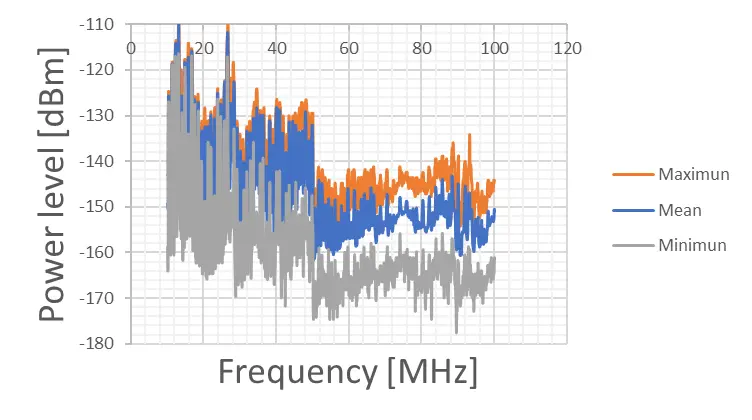
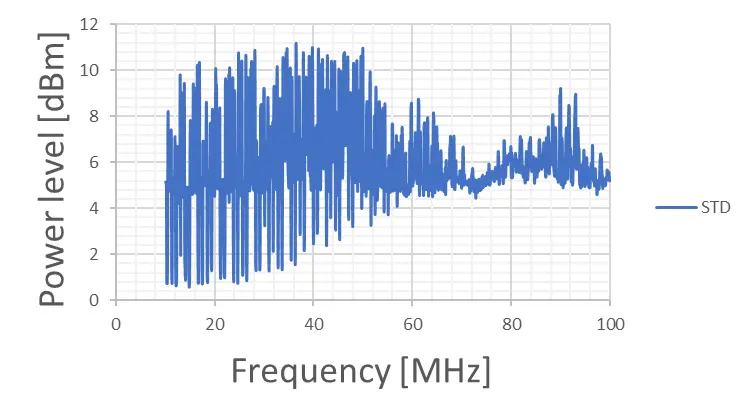
Example 1 - Noise spectrum Max, Mean and Minimum, as well as standard deviation at different frequencies for a noisy LED light.
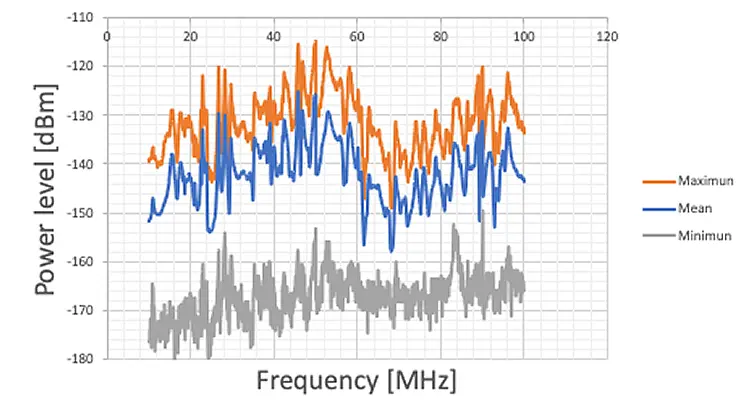
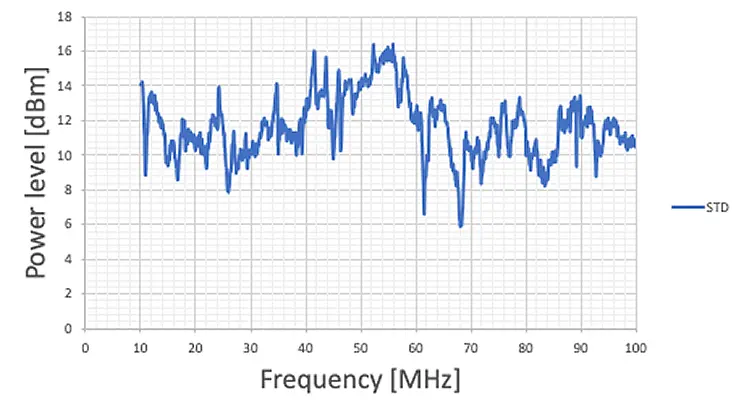
Example 2 - Noise spectrum Max, Mean and Minimum, as well as standard deviation at different frequencies for a different noisy LED light than the one in Example 1.
The Promptlink Noise Characterization Lab is equipped with advanced test and measurement instruments as well as software applications for measuring the spectrum and statistical parameters defining different types of noises and cataloging them for use in Data Labeling setup.
MER and Power Level Calibration for Noise Spectral Density
Power Levels and Modulation Error Ratio (MER) are critical parameters for noise and impairment localization, as well as for HFC network topology analysis. Utilizing these parameters allows for the derivation of Noise Spectral Density (NSD) across different network segments, providing key insights into noise origins within the network. However, the process faces a significant challenge due to the complex, non-linear relationships between NSD, power level, and MER, which vary across different modem brands, models, hardware versions, and firmware. To tackle this, Promptlink has developed a comprehensive test and measurement setup (MER/Power Level Calibration Lab) and is generating a calibration table for the MER and power levels of various modems.
Summary
The precisely configured cable plant in the Promptlink Noise Lab, coupled with the systematic characterization of noise sources and impaired network components, allows us to gather critical and difficult to generate labeled data. This data is invaluable for automated diagnostics and troubleshooting of cable plant issues, as well as for extracting its topology. The labeled data can be used to train Machine-Learning models for predicting and identifying:
- Location(s) where noise is entering the network
- Types of noise entering the network
- Location(s) where nonlinear distortion is originated in a HFC network
- Types of nonlinear distortion such as CPD or amplifier saturation
- Location(s) of failed or impaired network component(s)
- Type of failed or impaired network components
- Impact of noise and impairments on the network performance - troubleshooting priority
- Cable modem health versus performance - preventing unnecessary CM swaps
- Network Topology
As mentioned, the equipment used in this lab can be reconfigured to meet a virtually unlimited number of applications related to HFC Noise. Based on the needs and desires of customers, Promptlink can make this lab available for Data Labeling as well as Noise Characterization and other HFC Noise-related applications.